PhonSenticnet: Leveraging phonetics for concept-level sentiment analysis
November 2019 | ML, Publications
Problem
With the current trend of using microtext on social media platforms, eg - "luv" instead of "love", "dislyk" instead of "dislike" the task of extracting sentiments from text is becoming more difficlt with our systems not able to understand the meaning of these out-of-vocablary words.
Goal
To develop a phonetically augemented approach coupled with machine learning for transforming these words to their in-vocabulary forms for error-free sentiment analysis.
Role
Natral Language Processing
Machine Learning
Advisor
Dr. Erik Cambria
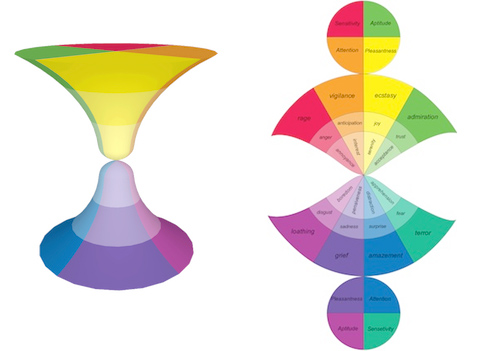
With the current upsurge in the usage of social media platforms, the trend of using short text (microtext) in place of standard words has seen a significant rise. The usage of microtext poses a considerable performance issue in concept-level sentiment analysis, since models are trained on standard words. This paper discusses the impact of coupling sub-symbolic (phonetics) with symbolic (machine learning) Artificial Intelligence to transform the out-of-vocabulary concepts into their standard in-vocabulary form. The phonetic distance is calculated using the Sorensen similarity algorithm. The phonetically similar invocabulary concepts thus obtained are then used to compute the correct polarity value, which was previously being miscalculated because of the presence of microtext. Our proposed framework increases the accuracy of polarity detection by 6% as compared to the earlier model. This also validates the fact that microtext normalization is a necessary pre-requisite for the sentiment analysis task.
The table below shows some sentences with their detected polarity before and after microtext normalization:
–> Link to Publication.